Rebeca Figueiredo
Identifying disease-specific mechanisms using transcriptomics data through co-expression networks and pathway knowledge
Master’s student, Rebeca Figueiredo, discusses her recently submitted work on overlaying pathway knowledge with co-expression networks to identify disease-specific mechanisms.
Despite the exponential growth of biomedical data in the last decades, we are still far from understanding the function of every gene in a living organism. Nevertheless, we can assign specific biological functions to thousands of protein-coding genes in the human genome.
This allows us to create complex interactions between groups of genes, proteins, and other biomolecules to indicate the normal functioning of the cell. By acquiring knowledge of these interactions into a human interactome network, we can decipher the molecular mechanisms which cause system-wide failures that can lead to disease through networks.
Bridging disease signatures with pathway knowledge
Our goal is to use a systematic network-based approach that builds a bridge between disease signatures and pathway knowledge to better understand human pathophysiology. We collected thousands of transcriptomic datasets from over 60 diseases and explored expression patterns observed in their corresponding co-expression networks to the network representing “healthy” reference samples and an interactome network with pathway knowledge at three different scales. At each of these scales, we have investigated which proteins, subgraphs, and pathways could be associated with both disease-specific and shared mechanisms. Firstly, at the most broad scale, we identify the most and least common proteins in these diseases and evaluate their consistency against the interactome as a proxy for their prevalence in the scientific literature. Secondly, we overlay both network templates to analyze common correlations and interactions between proteins across diseases. Thirdly, we explore the similarity between patterns observed at the disease level and pathway knowledge to identify pathway signatures associated with specific diseases and indication areas. Fig. 1 illustrates our methodology and steps in our systematic analysis.
Application and in depth investigation
Our analysis has enabled us to globally evaluate the consensus between disease-specific transcriptomics data and an integrative human interactome network.
In a case scenario, we have demonstrated how our approach can be used to investigate the role of a specific pathway in a disease-specific context. Our pathway-level analysis (Fig. 2) points out that the interactions in the pathway for long-term potentiation (LTP) yields the highest similarity to the schizophrenia co-expression network. In an in-depth investigation between these, we overlaid the disease network with the pathway and found major common interactions between the two examined the differential expression of the involved proteins in schizophrenia, and highlighted new interactions from those that were found to be highly co-expressed in schizophrenia but are not documented in the LTP pathway.
If you are interested in our work, our pre-print can be read here and publication soon to come.
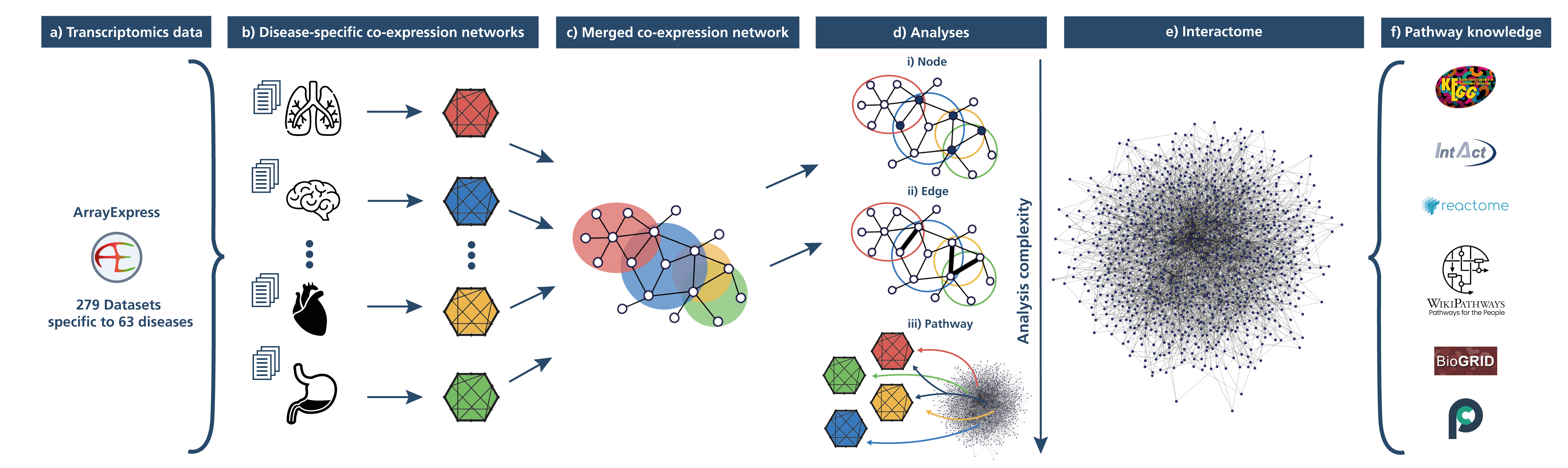
Figure 1: Schematic illustration of the methodology. Transcriptomic datasets were acquired from ArrayExpress (a) and grouped into distinct diseases to generate disease-specific co-expression networks (b). A comprehensive protein-protein interactome network was built (e) from an ensemble of six pathway and interaction databases (f). A series of analyses were then conducted on the disease-specific co-expression networks (d), by leveraging pathway knowledge and the interactome network.
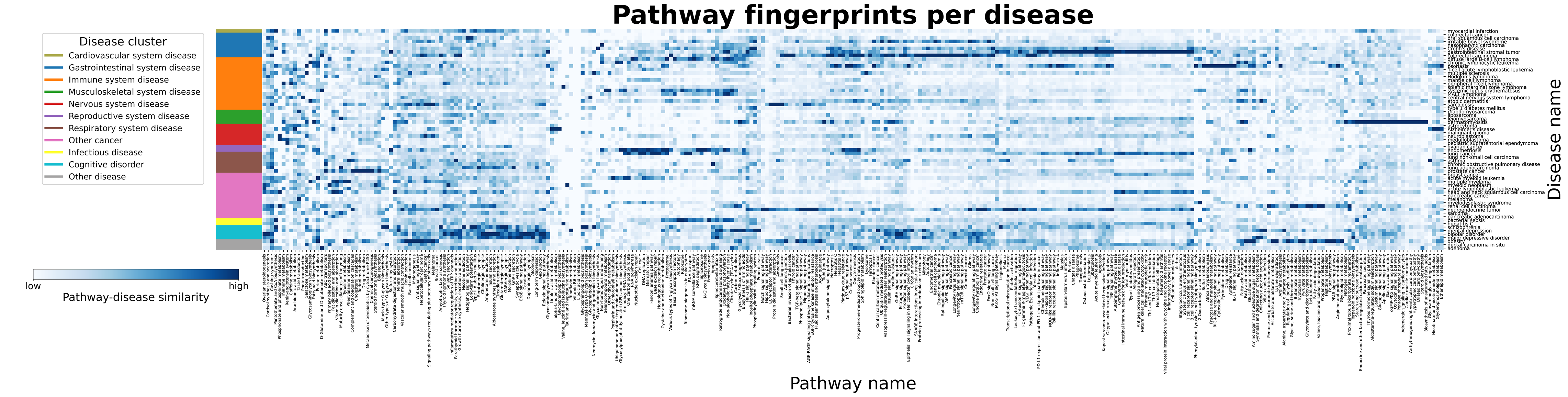
Figure 2: Mapping disease-specific expression patterns with pathway knowledge via network similarity. The heatmap illustrates the consensus similarity between pathways and disease co-expression networks. Lighter values correspond to a lower similarity and darker values correspond to higher similarity. A high quality version can be seen here.