Bruce Schultz
Harmonization of multimodal knowledge
Bruce Schultz discusses his recent paper on synthesizing data and information in the context of COVID-19 in order to predict drug repurposing candidates.
Thanks to collaborative efforts of research groups around the world, there is a vast quantity of data available pertaining to both SARS-CoV-2 and COVID-19, and how they affect us at the molecular level. However, these resources store their collected information in several, often incompatible formats, thereby preventing one from assembling a complete picture of the knowledge available. As drug repurposing is the most viable method for quickly identifying new therapeutics for treating diseases, we integrated public data and experimental results into a comprehensive network of mechanisms involved in COVID-19 in order to predict new candidate compounds for treating this widespread disease.
Several established models and interaction databases pertaining to COVID-19 are publicly available online, though not one of them covers all aspects of the virus or disease. In order to integrate these resources as well as the latest experimental data into a single network, each individual interaction was first encoded into Biological Expression Language (BEL). This language is ideally suited to capture the “cause-and-effect” relationship of molecular interactions in the context in which they occur. Once converted, these interactions were loaded into a graph database using e(BE:L) and enriched with additional metadata, including drug-target information from DrugBank, ChEMBL, and PubChem, to generate the COVID-19 PHARMACOME. The COVID-19 PHARMACOME is a compilation of drug-target mechanisms in the context of COVID-19 pathophysiology. Using known combination treatments to establish a pattern, we were able to predict new repurposed drug combinations from the COVID-19 PHARMACOME and experimentally validate our findings.
Though we have used the COVID-19 PHARMACOME for identifying new drug repurposing candidates, the network is annotated with a wide spectrum of data from a variety of resources. Users can apply their own algorithms and explore the mechanisms governing SARS-CoV-2. The COVID-19 PHARMACOME is available to the public at http://graphstore.scai.fraunhofer.de. Users can freely access the available integrated data and explore the network. For a more user-friendly experience, we have also created the Biological Knowledge Miner (BiKMi), a web interface that has a collection of predefined tools for querying the graph database itself.
The COVID-19 supergraph integrates drug-target information to form the COVID-19 PHARMACOME.
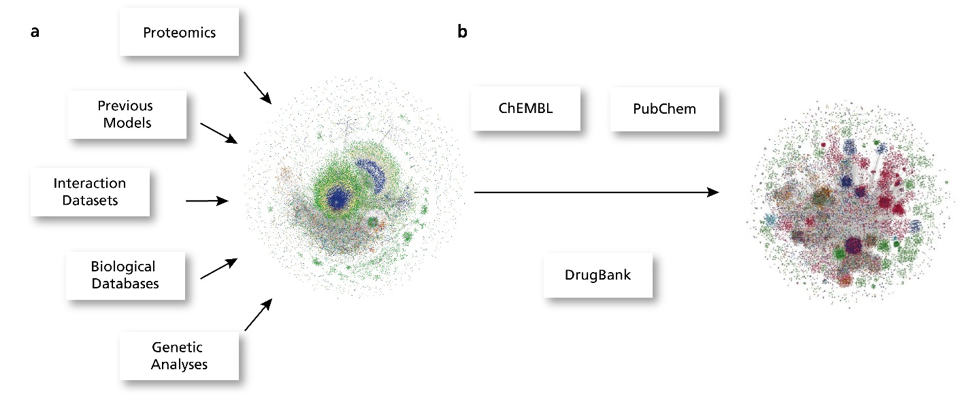