Tamara Raschka
Variational autoencoder modular Bayesian networks for joint modeling of clinical data and molecular disease mechanisms in Alzheimer's disease
Tamara Raschka writes about here recent work on an AI strategy to quantitatively model biological mechanisms for systems medicine in Alzheimer’s.
Mechanistic modeling of biological systems is a key for better disease understanding and for supporting the identification of potential drug targets. A typical approach taken in systems medicine is mathematical modeling via differential equation systems. However, formulating such models requires detailed knowledge of relevant biochemical processes, and fitting of the systems does need time resolved data and the possibility to perform control experiments. All this does not exist in many diseases, such as Alzheimer’s Disease.
In our work we addressed this problem by employing our recently published Variational Autoencoder Modular Bayesian Network (VAMBN) approach [1], which is a hybrid of variational autoencoders and modular Bayesian Networks. We trained VAMBN on combined clinical and patient level gene expression data while using incomplete prior knowledge about disease mechanisms in form of a graph. Therefore, combining the data-driven AI approach VAMBN with knowledge graphs enabled us to build on existing knowledge while still being able to identify new links between the different components of the network. Modules of the modular Bayesian Network were obtained through a statistical overrepresentation analysis of Alzheimer Disease specific mechanisms within clusters of the knowledge graph. Our approach resulted into a model that could well explain patient data from several studies. It proposed a path between CD33 and cognition through the Ubiquitin degradation pathway.
Additionally, with that model in hand, we simulated a knock-down of CD33, showing significantly increased cognition (MMSE) scores (see Figure 1), meaning that patients with therapeutically inhibited CD33 could have better cognition.
Results from this simulation are highly overlapping with cell line gene expression experiments, hence supporting the findings from our model.
All in all, our quantitative modeling approach could thus help to select promising drug targets in neurological disorders.
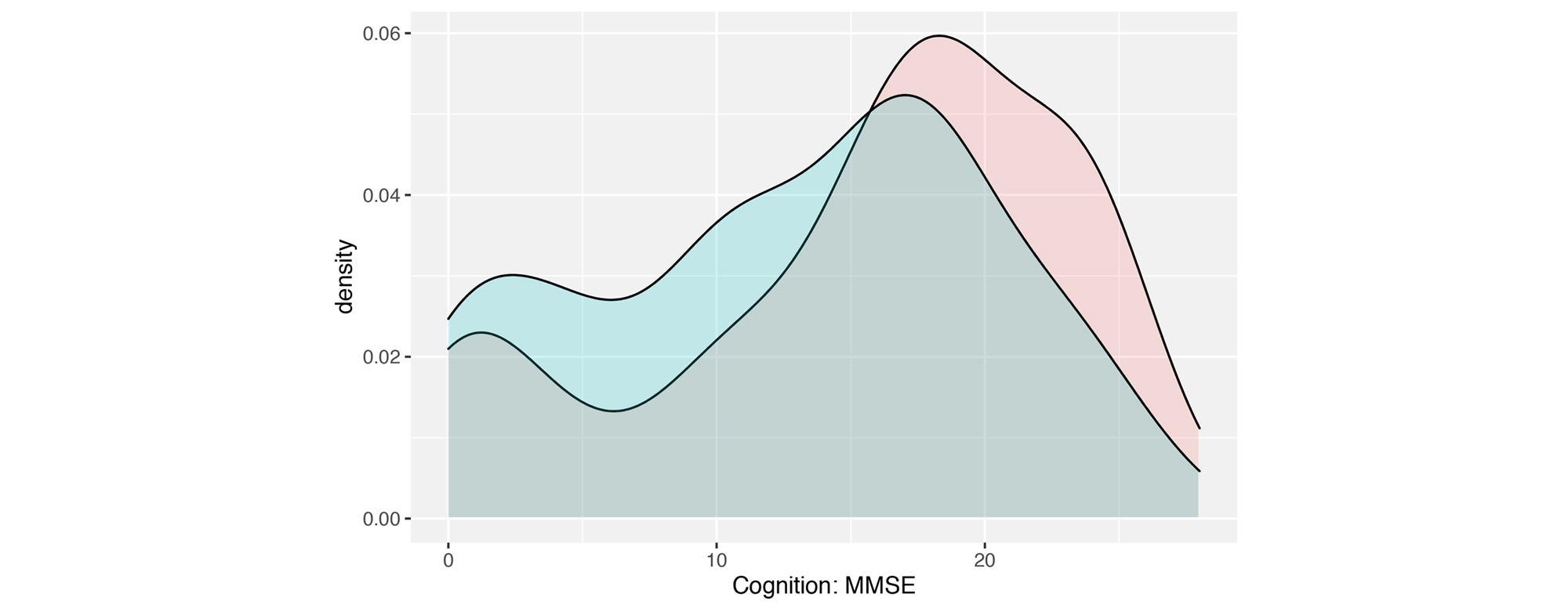
References:
[1] Gootjes-Dreesbach, L., Sood, M., Sahay, A., Hofmann-Apitius, M., & Fröhlich, H. (2020). Variational Autoencoder Modular Bayesian Networks for Simulation of Heterogeneous Clinical Study Data. Frontiers in big Data, 3.